In the past decade, practitioners have seen a dramatic increase in data availability. The emergence of big data has significantly influenced how data is collected and used. This abundance of data has enabled new kinds of analyses that were not possible. However, the volume and complexity of these datasets has left many without a clear strategy for their use.
Consequently, practitioners are challenged with not just choosing relevant data sources but also turning them into actionable insights. This task is made more challenging as the global data volume expands. Although each data source offers unique insights, often no single source can answer all the complex questions that planners and policymakers face.
Awash in a sea of data, practitioners seek guidance on selecting the right big data sources, addressing biases, and integrating big data with existing survey and model frameworks. Addressing these challenges requires a deep understanding of travel behavior, the ability to assess various data sources, and the skill to turn these insights into practical tools and decisions.
This article outlines the evolving landscape of passively collected data and discusses the utility of various data types while proposing a forward-thinking approach to their selection, application, and integration.
The Value of Passively Collected Data
The term “passively collected data” might seem complex, but it just means collecting data without the user's active involvement. This type of data focuses on how it's collected, whereas big data is about the data's characteristics and the challenges it presents. Big data is known for its three Vs: volume, velocity, and variety. It involves large amounts of data, arrives rapidly, and includes different types of data (structured, unstructured, and semistructured).
Each source has its biases and limitations, complicating their optimal use. Smartphone ownership in America has jumped to 90% today from 35% in 2011, according to the Pew Research Center, making these devices a major player in the big data revolution. Yet, the quality of data from location-based services (LBS) started to decline in 2022, leading many providers to shift to connected vehicle data (CVD) instead. CVD and synthetic datasets further contribute to the big data ecosystem's increasing diversity and complexity.
The breadth of these data sources provides deep insights into travel patterns and behaviors, which transportation agencies can use for planning. By using the entire spectrum of data, including passively collected data, practitioners can improve the accuracy of their forecasts. This integration reveals essential insights into new areas, such as equitable access to mobility services and the adoption rates of technologies like electric vehicles (EVs), offering a detailed perspective on societal trends and emerging technologies.
The Pros and Cons of Big Data
Big data complements traditional surveys by uncovering wide-ranging travel patterns that smaller datasets may not reveal, such as long-distance trips, visitor movements, and commercial vehicle flows. This broader view is a key benefit of big data, providing a complementary and more detailed and nuanced understanding of mobility.
Despite its advantages, navigating the big data landscape presents challenges. Practitioners often struggle to select and use data sources effectively, an issue that arises when they realize—usually after acquiring the data—that no single dataset can meet all of an agency's needs. This highlights the need for strategic planning in choosing data sources from the start.
Additionally, integrating big data into existing frameworks, like travel surveys or model development, poses a major barrier to its adoption. The initial enthusiasm for accessing vast datasets has been replaced by the complex challenge of effective integration, which often involves a steep learning curve. This complexity can discourage users from moving past initial data acquisition or confine them to using the data for just one application.
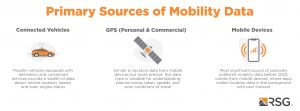
The primary sources of mobility data include connected vehicles, GPS (personal & commercial), and mobile devices.
An Evidence-Backed Approach to Mobility Data Analytics
An evidence-backed approach to mobility data analytics requires carefully integrating big data, from collecting travel survey data to developing travel models. This process shouldn't just focus on the technical aspects of integrating big data, which is only the beginning. It also needs to prioritize using high-quality data and proving tangible improvements in forecasting accuracy, which can inform strategic planning and policy decisions throughout an organization.
As experienced advisers in data collection and modeling, our experts have worked with all the major big data sources available. From these experiences, we've gained valuable insights that can assist clients in navigating the big data vendor landscape and addressing key planning and policy questions for their constituencies. When choosing a big data source to achieve strategic objectives, practitioners should keep three important considerations in mind.
1. Peek Under the Hood
Practitioners aiming to incorporate big data into their travel surveys or model development workflows can encounter challenges in matching their expectations with reality at the start of projects. This gap underscores the need for thorough screening and evaluation criteria to ensure selected big data sources add value to projects.
To ensure the selected data source aligns with the project's unique goals, it's crucial to identify information gaps and understand specific needs. We guide our clients in creating a detailed list of questions (see below) for potential data vendors, focusing on factors that ensure the project team has a common understanding of the significance of data relevance and quality. This step is critical in guaranteeing the vendor's data meets the project's precise requirements, laying a strong basis for informed decision-making.
2. Evaluate Reasonableness and Understand Biases
After selecting a data provider, practitioners should evaluate the reasonableness of the big data source they have selected. To ensure the big data can match the proven accuracy and explanatory power of survey data, practitioners should conduct a comprehensive assessment of precision, completeness, and representativeness.
Big data offers valuable insights but can also contain biases, such as sampling, location, behavioral, temporal, technology, and algorithmic biases in synthetic data, which may even be unknown to the data providers. To manage these biases, it's important to scrutinize data collection methods, ensure vendors' algorithmic transparency, and continuously validate forecasting models with real-world outcomes.
To ensure applicability and accuracy, we employ advanced statistical techniques and tools designed to identify and correct these biases. The types and applications of these tools vary by project and are tailored to a client’s specific needs and use cases.
3. Consider How You Plan to Use the Data
Understanding the objectives for data utilization is crucial. Do you plan to leverage the data to augment existing data sources across multiple applications, or is your goal to address a narrow policy question? This determination is vital as it shapes the approach to data exploration and analysis.
Data mining is a key technique in the context of big data, involving the detailed exploration of large datasets to discover hidden patterns, correlations, trends, and valuable insights that are not evident through casual observation, due to the data's vast volume and complexity. Utilizing sophisticated techniques from machine learning, statistics, and database management, data mining aims to extract actionable knowledge that might be missed by manual analysis.
When practitioners have access to both travel survey data and big data, there is a unique opportunity to blend the rich, self-reported insights from travel surveys with the more extensive, but typically less-detailed insights from big data. RSG has applied this methodology to an innovative augmented mobility data analytics project in Florida. This work gave the client comprehensive insights into regional travel behaviors, highlighting significant shifts post-pandemic, such as changes in downtown activity, public transit ridership, and work-from-home trends.
Our strategy in utilizing mobility data analytics is to convert raw data into a structured format tailored to our clients' modeling requirements, leveraging our expertise as both developers and users of models. This targeted approach guarantees that the integration of big data enhances value effectively and accurately, aligning with client expectations.
Longer-Term Implications for Transportation Planning
Thoughtful and methodological integration of passively collected data sources into the travel model development process represents an exciting and promising frontier in transportation planning. Our team's experience has shown that when these data sources are used in conjunction with traditional traffic counts and other empirical data, the outcomes are greatly enhanced.
To meet the expectations of various stakeholders, evaluating each model should include assessing how the addition of passively collected data affects its performance. This process entails a meticulous comparison of model forecasts against real-world data, typically from travel surveys, to evaluate precision, reasonableness, and representation. This detailed examination by the project team helps ensure the models are not just theoretically sound but also reflect real trends and behavioral changes accurately.
Successfully integrating passively collected data into travel models requires a wide range of skills and a deep understanding of each data source's benefits and drawbacks. The primary goal in this process is to improve the model's capacity to forecast future conditions accurately, reflecting present circumstances and predicting future changes. Attaining such precision significantly enhances the model's predictive power, making it better equipped to adapt to changing transportation trends and demands.
Explore the Potential of Big Data through Mobility Data Analytics
Using big data as part of the transportation planning process can help practitioners understand and forecast travel behaviors. By delivering data at a greater volume and scale to complement the accuracy and precision of survey data, big data can also broaden the utility of travel models.
Our team of experts has evaluated and used big data from all the major data providers and routinely works with these sources to deliver actionable insights for our clients. More importantly, our combined expertise in travel surveys, travel model development, and mobility data analytics means we can help our clients navigate the complexities of big data while more effectively serving their constituencies.
Reach out to one of the members of our team to learn how we can help you select the appropriate data source for your project needs.