The monetization of passively collected data and data not traditionally collected for transportation research has transformed how agencies approach mobility challenges. The scale of these datasets enables new analytical approaches and insights into travel behavior and mobility patterns. Since their early applications, big data sources in transportation have evolved dramatically. Vendors frequently revise their methodologies, and new data sources continually emerge while others fade. This makes it challenging for agencies to identify dependable, actionable insights confidently.
For organizations using these data sources, the stakes are high—integrating mobility data effectively can unlock more accurate planning and support data-driven policy decisions. However, navigating the technical intricacies of the multitude of data options that tell different stories that are often inconsistent remains a challenge. How can agencies confidently select, evaluate, and apply these data sources? This article presents an evidence-based framework for choosing transparent, high-quality big data solutions aligned with long-term planning goals.
Understanding the Big Data Landscape
The rapid evolution in big data sources, privacy standards, and vendor practices means that a one-size-fits-all approach around big data use is no longer feasible for agencies. Practitioners face a market where data reliability, coverage, and accessibility vary significantly across sources.
“Passively collected data” refers to data gathered without requiring active participation from the user, such as data captured through mobile devices, connected vehicles, or sensors. This type of data distinguishes itself by the method of collection.
“Big data” refers to the sheer volume, speed, and diversity of data. Big data includes large, fast-arriving datasets in structured, unstructured, and semi-structured forms. For clarity, this article uses “big data” to describe these datasets.
To help agencies make sense of this complexity, we highlight key trends shaping the most commonly used transportation data sources.
Location-Based Services Data
Location-based services (LBS) data, often collected from mobile phones, has traditionally been a mainstay for understanding travel patterns. However, several significant shifts have reduced its reliability. In 2021, Apple and Google (Android) implemented privacy measures, such as App Tracking Transparency, which allow users to limit third-party access to their location data. This change led to sharp reductions in available LBS data points, complicating longitudinal travel behavior tracking and causing gaps that reduce its utility for travel demand modeling.
Beyond technology, broader developments have further impacted LBS data. The US Supreme Court’s 2022 decision in Dobbs v. Jackson Women’s Health Organization created ripple effects in the data world, heightening privacy concerns around sensitive travel data. Several major data aggregators now restrict location tracking near healthcare facilities and other sensitive areas. International factors, such as Google’s restriction on SafeGraph, an LBS provider with foreign investor ties, have added further challenges. These developments mean that LBS data, once essential to mobility analysis, may no longer provide the consistent coverage required for many transportation planning applications.
Connected Vehicle Data
As LBS data’s reliability has declined, connected vehicle data (CVD) has emerged as a promising alternative. CVD offers detailed metrics from in-vehicle sensors, such as speed, location, and operational data, providing a granular view of vehicular travel patterns. However, CVD data primarily represents newer, high-end vehicles equipped with connectivity features, meaning it likely skews toward higher-income individuals. This bias can affect data representativeness, especially in regions with lower rates of new vehicle ownership.
Additionally, CVD only tracks vehicles in motion, excluding inactive phases and nonvehicular travel. While useful for auto-centric analyses like traffic flow, speeds, and congestion, CVD is not useful for comprehensive studies that require insights into pedestrian, biking, or transit patterns. As a result, CVD is valuable for roadway-focused studies but limited as a stand-alone data source for broad transportation planning.
Aggregated GPS Data
Aggregated GPS (AGPS) data, a source that has found new relevance for transportation planning applications, offers a unique blend of device navigation apps, traditional mobile data apps, and in-vehicle navigation apps. The multiple sources result in a highly representative sample with strong penetration rates across road sizes and regions, offering a broader population sample than CVD and one more in line with LBS. Unlike CVD, AGPS can capture a variety of travel modes, not just vehicular data. However, AGPS is relatively untested in the transportation planning space and is often focused on roadway segment travel rather than land-use origin-destination patterns, which can limit its applicability for certain planning purposes. While promising, AGPS likely requires further validation to establish its reliability across diverse transportation scenarios.
Examples of Multisource, Evidence-Backed Approaches to Mobility Data Analytics
Given the volatility and complexities of the big data landscape, RSG’s approach to mobility data analytics involves working with clients to combine diverse data sources as needed and validate data quality. By adopting a multisource, evidence-backed approach, we help clients develop a clear, actionable picture of travel behavior, even in challenging contexts. This process aligns with our commitment to providing high-quality, defensible insights that support informed, data-driven decisions.
Blending Data for Comprehensive Insights
For the Tampa Bay Regional Travel Survey, understanding travel patterns in a high-tourism region required a multisource approach. Given the region’s dynamism, relying on a single data source could have missed important insights into visitor travel behavior. To address this, we combined Replica data, LBS data, and a visitor-specific travel survey using rMove™. This combination allowed us to cross-validate data, capture seasonal visitor fluctuations, and identify key origins for out-of-state travelers.
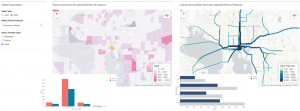
RSG worked with the Florida Department of Transportation (FDOT) District Seven (D7) and several metropolitan/transportation planning organizations to understand travel patterns in a high-tourism region required a multisource big data approach that combined Replica data, LBS data, and a visitor-specific travel survey using rMove.
This multisource approach reduced the limitations of each dataset, resulting in a single data product that is more than the sum of its parts. For example, Replica data provided a solid baseline but underreported visitor traffic due to its limitations in cross-regional ID tracking. Supplementing it with empirical data from rMove addressed this gap, resulting in a more accurate, comprehensive view of visitor behavior in the region.
Big Data Product Evaluation
The Northeastern Indiana Regional Coordinating Council (NIRCC) needed data for planning and model development but was uncertain about which data sources would best meet its needs. Our team led a pre-evaluation phase to assess sample data from multiple vendors, including StreetLight, AirSage, INRIX, Replica, and LOCUS. This preliminary analysis allowed us to examine metrics like trip purpose, mode, distance, and sample rate, informing the strategic selection of data sources for a future study.
This evidence-based pre-evaluation prevented costly missteps, ensuring that the selected data sources aligned with NIRCC’s planning objectives. Our upfront multisource evaluation approach identified potential biases and validated metrics, instilling greater confidence in the chosen datasets.
Validating and Refining Data with Survey Comparisons
The Tahoe Regional Planning Agency (TRPA) aimed to understand post-COVID travel patterns in a context where seasonal visitors and commuting service workers heavily impact local congestion. Preliminary discussions revealed that previous data sources, including Replica and LOCUS, did not align with observed traffic counts in high-tourism areas.
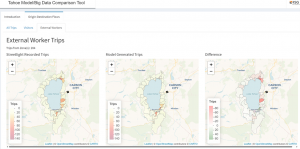
RSG worked with the Tahoe Regional Planning Agency to leverage our relationships with big data vendors to develop a custom dataset that categorized trips by residents, employees, and visitors. We then developed visualizer that shows how the observed data differed from what the model was forecasting.
By comparing big data with empirical survey data, our team helped TRPA determine that Replica, while imperfect, was the better starting point for refining mode-of-travel shares. Building on this insight, we developed a custom dataset from StreetLight LBS data that categorized trips by residents, employees, and visitors. This tailored solution provided a more accurate, defensible foundation for TRPA’s congestion and travel demand modeling.
Layering Data to Capture Complex Local Travel Patterns
The Napa Valley Transportation Authority (NVTA) sought to update its travel behavior studies to better understand complex travel dynamics, including commuter and service worker patterns along congested corridors. Given the unique socioeconomic profile of the Napa Valley workforce, we worked with StreetLight to identify data sources that would minimize sampling biases, particularly those skewed toward higher-income, vehicle-owning populations.
By layering multiple data sources, including LBS, AGPS, and a supplemental employer survey, we are creating a dataset that accurately represents diverse population segments. This combined approach will enable NVTA to examine travel patterns for lower-income, service-sector workers who may otherwise have been underrepresented in a single-source data approach.
Tailoring Freight Data for Fleet Electrification Planning
Prologis, a global leader in logistics real estate, needed to assess fleet vehicle travel patterns to support its electrification infrastructure planning. Using Geotab’s telematics data from light-, medium-, and heavy-duty trucks, our team analyzed travel corridors, trip lengths, parking behavior, and logistics nodes. Unlike traditional travel studies, this analysis required data that could capture intermediate stops, trip-chaining patterns, and truck classifications—key for Prologis’s infrastructure siting.
By customizing data collection to meet freight-specific needs, we equipped Prologis with analysis that extended beyond standard origin-destination patterns, enabling data-driven decisions for EV infrastructure placement based on real-world travel behavior.
Increasing Confidence in Big Data Decisions
For agencies incorporating big data into transportation planning, RSG encourages proactive questioning to vet data source viability. Key questions include:
- Data Source and Collection: What is the data’s origin, and what collection methods are used? This impacts applicability for various planning scenarios.
- Sample Rates and Representation: What’s the sample rate, and how representative are the data? Knowing biases is essential for understanding demographic representation.
- Data Cleaning and Enhancement Methods: How have the raw data been cleaned, adjusted, and manipulated to produce the product?
- Privacy and Compliance: How does the vendor manage privacy concerns? Compliance with recent policy changes ensures ethical, legal data use.
- Data Quality Control: What quality control measures are in place? Reliable documentation helps ensure the data’s defensibility.
- Coverage and Gaps: Are there known data gaps? Knowing data limitations helps evaluate source suitability for specific needs.
RSG’s multisource integration approach ensures that data selection is strategic and transparent and supports long-term planning. With expertise in rigorous data analytics, RSG guides agencies through complex data environments to produce insights that drive impactful, data-driven decisions.
As the big data landscape continues to evolve, RSG remains committed to staying up to date on the state of the market and leading clients through every stage of their data journey—from evaluation or collection to integrated analytics and model development. For agencies seeking confidence in data-driven decision-making, RSG’s approach provides a pathway through big data’s uncertainties.
Ready to approach mobility data analytics with confidence? Contact us to explore how our expertise can provide clarity and actionable insights for your organization.
····························
About the Authors
Kevin Johnson is a transportation planner with expertise in combining the application of travel demand models with data derived from passive mobility data analytics (“big data”) to deliver future-focused client solutions. Kevin has contributed to projects seeking to collect big data using cellular, GPS, and location-based services technologies. In addition, he has applied his expertise in these areas to work with warehouse and logistics companies to help them plan proactively for future network deficiencies.
Kevin will be presenting this topic at the 2025 Transportation Research Board Annual Meeting on Monday, January 6 from 1:30 p.m. to 3:15 p.m. ET during Poster Session 2158.
Kevin will also be sharing insights into RSG’s use of Geotab data as part of our approach to mobility data analytics during the Geotab Connect conference in Orlando, Florida, from February 25 through February 27.
Stephen Lawe has worked in the transportation forecasting and planning industry for over 35 years. He focuses on thought leadership, working with clients to bring about transformative change, and mentoring the next generation of leaders. He serves as a technical adviser on select projects and leads research to understand the challenges facing RSG's clients, including into issues surrounding the use and selection of big data products.