Travel model development involves creating sophisticated simulations of transportation systems to predict travel patterns and behaviors. These simulations enable planners and policymakers to make informed decisions that enhance communities’ mobility and improve outcomes.
RSG's experts develop travel models, specializing in activity-based models that provide a more nuanced view of travel and better simulate real-world activities and travel patterns. Joel Freedman, a nationally recognized leader in advanced travel demand forecasting models, has extensive experience in travel survey design, model estimation, software implementation, validation, and application. After earning his master’s degree in urban planning from Portland State University, Joel worked for three years at a metropolitan planning organization (MPO) before transitioning to consulting on travel modeling projects.
In his role as Principal at RSG, Joel helps develop travel demand models for MPOs and state departments of transportation across the United States. Since joining RSG, he has been an influential architect of ActivitySim, working closely with clients and consortium members to advance the state of the practice for activity-based modeling.
We connected with Joel to talk about travel models, ActivitySim, and the benefits clients reap from RSG’s integrated data collection and modeling capabilities.
Why are travel demand models so central to transportation planning?
Travel demand models are important tools for transportation agencies. Several types exist, with the most common being trip-based models, also known as four-step models. These have been in use for decades, named for their sequence of operations: trip generation, trip distribution, mode choice, and network assignment. Activity-based models, which are somewhat newer, offer higher demographic, spatial, and temporal resolution, allowing for a more detailed exploration of the types of policy and planning questions increasingly faced by planners nowadays.
Some agencies have more limited uses for their travel models, such as traffic projections at intersections, where sophisticated models may not be necessary. However, if an agency is dealing with more complicated questions, they may want to consider more sophisticated models such as activity-based models. Travel models help their users meet federal and state requirements and effectively prioritize transportation projects and federal funding. Without such models, planners would have to rely on less transparent or rigorous methods. Travel models provide an objective basis for evaluating transportation investments, moving the discussion to quantifiable debates about the assumptions within the models. This transparency helps us identify and address inherent biases and assumptions, fostering more informed critiques and continuous improvements in modeling practices.
Regulations such as the Clean Air Act amendments often require the use of travel demand models to evaluate air quality by regions in “non-attainment” of specific pollutant standards. For instance, the US Environmental Protection Agency mandates the use of a tool called MOVES (MOtor Vehicle Emission Simulator) that integrates with travel models to measure potential emissions created by each package of transportation investments considered by the region. Additionally, state laws and agencies like Washington State’s Growth Management Act and the California Air Resources Board require analyses to ensure that proposed new developments do not increase per capita vehicle miles, which are closely linked to rising emissions that contribute to climate change.
What is the primary motivation or reason for a state or region choosing to develop a travel demand model and what types of models are in use?
At its core, a travel model estimates demand based on growth assumptions about population, employment, and changes in the transportation network. While alternative methods like trend analysis or simple back-of-the-envelope calculations exist, travel models provide a comprehensive accounting system for these factors. The more sophisticated the model, the more accurate its forecasts. Most large metropolitan areas employ a travel demand model, although the prevalence of statewide models varies.
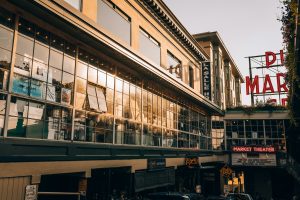
According to the Travel Forecasting Resource, as of 2016, 34 states had an operational statewide model, 4 states were developing models, and 12 states did not have an operational statewide model.
The types of models in use at the state and regional levels include trip-based, activity-based, and hybrid models, which combine elements of both. The absence of a statewide model in some heavily populated states may stem from respect for home rule and the existence of models/forecasts from MPOs. However, the lack of a statewide model does not imply that a state lacks a rigorous and well-designed planning process. For example, Washington State, despite not having a statewide travel model, engages in extensive statewide planning. They have enacted the Growth Management Act, managed land-use restrictions, state highways, and a ferry system, used custom travel models for specific projects, and relied on regional models when necessary for project planning and policy analysis.
How has ActivitySim and the work undertaken by the consortium expanded access to the forecasting capabilities of activity-based models, and what excites you most about its future?
RSG has been a leader in the activity-based modeling space for decades, and in that time ActivitySim has become an increasingly crucial tool in our toolkit as modelers. The collaborative nature of the ActivitySim consortium offers a compelling option for agencies that might be hesitant to adopt a new paradigm of modeling. Being part of a consortium where multiple agencies use the same platform can ease that transition, as agencies benefit from the collective advancements and experiences of their peers. This collaborative approach not only enhances the accessibility of advanced forecasting capabilities inherent in activity-based models but also fosters a supportive network for shared learning and innovation. What excites me most about its future is the potential for widespread adoption and continuous improvement, which are driven by the consortium’s collective insights and efforts.
How does having data collection and travel demand modeling capabilities under one roof benefit our clients and ensure the strength of their data pipeline?
At RSG, having both data collection capabilities and tools, such as rMove™, and travel demand modeling capabilities under one roof provides substantial benefits to our clients and ensures the accuracy and relevancy of their data pipeline. We regularly meet internally to discuss these projects, ensuring that we're processing and expanding the data accurately. Personally, I am routinely engaged in our household travel survey projects as a technical adviser and expert, which benefits our clients in several ways.
For instance, we recently discussed our survey expansion methodology with a state department of transportation. They needed assurance from our modeling team that we could provide survey expansion weights that would match each of their state’s MPO boundaries. This conversation benefited all parties involved, and we confirmed our capability to meet their data expansion requirements. Our clients feel more at ease knowing that someone from the data collection side understands modeling practices and can advocate for data that aligns with travel demand modeling needs.
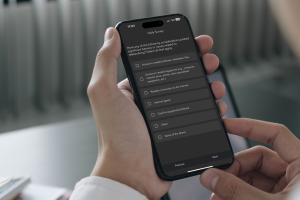
rMove is RSG's location-aware market research app. Data collected using rMove can be combined with other mobility data sources to deliver more accurate insights to clients.
Problems can arise during model development if the data collection team lacks awareness of how the data are used in modeling. This can lead to delays and cost overruns. For example, discarding a significant portion of the data may be necessary due to quality issues or lack of completeness, or the data may require re-expansion or further processing to ensure a more accurate representation of travel. When it comes time to use the data collected in a model, such situations might require significant additional work to rectify issues, and in the worst cases, the data might not even be usable for model development.
There is significant synergy in having both data collection and model development under one roof, and this integration works both ways. Our expertise in modeling enhances our data collection efforts. Working on the household travel survey projects helps us understand the data and the region more comprehensively. We learn what issues are important to the client and can design our surveys with those specific issues in mind. For example, we heard directly from another state DOT client that bike travel, e-commerce, and changes in vehicle miles traveled (VMT) per capita were important for them to understand, so we tailored our survey approach to capture these elements. Our team’s agility and foresight also help us think about how to address these issues in our models.
Apart from a ground-up redevelopment process, what options are available to agencies looking to update parameters to ensure their models, trip-based or activity-based, remain accurate predictors of travel behaviors?
The pandemic has significantly altered travel behavior, including changes in commuting patterns. To ensure that both trip-based and activity-based models remain accurate predictors of travel behavior, agencies have several options beyond a complete redevelopment of their models. Analysts can use census data to infer changes in work travel. However, this method does not account for changes in nonwork travel—for example, running personal errands while working from home—so agencies might look to borrow modeling parameters from regions with post-pandemic data or use passively collected data, if available. Additionally, traffic counts and transit ridership data can be crucial for recalibrating and revalidating model parameters. Although conducting a new household travel survey would be ideal, these alternative data sources can prove useful to update models to reflect changes brought about by the pandemic and technology between household travel survey projects.
Drawing on our team’s extensive mobility data analytics experience, RSG frequently incorporates passively collected data sources during our travel model development process. These data sources inform both internal and external flows through the region or help us analyze freight travel using sources like ATRI or Geotab data. We also employ passively collected data as a validation check on passenger trip tables. For example, passively collected data helped our team understand significant changes in the region for a regional planning agency whose last household survey was conducted about 20 years ago.
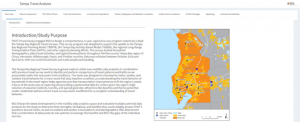
RSG worked with FDOT D7 to develop a transparent and methodologically sound framework that combined traditional travel survey data collected using rMove with data from Replica and other location-based services (LBS) data sources. Our interactive storyboard made the study’s voluminous data accessible and understandable to stakeholders.
What are corridor studies, and how do they relate to RSG’s travel model development work?
Corridor studies focus on specific areas of a transportation network to understand and improve traffic flows and congestion, evaluate alternative modes of travel, or other options such as high-occupancy lanes and pricing. Practitioners are increasingly using dynamic traffic assignment (DTA) models to analyze such alternatives. Traditional travel models often use static equilibrium models, which do not consider factors like departure times or queuing. However, DTA changes this by incorporating departure times and simulating how vehicles interact on the network in time segments. This method offers a more realistic representation of traffic dynamics, addressing a static model's limitations.
The issue with DTA lies in computational challenges associated with representing the increased network and vehicle resolution of these models and dealing with the methodological differences among the various DTA packages available to planners. At RSG, we are particularly interested in DTA because of its potential to significantly enhance decision-making for our clients. By providing more accurate information on traffic flows and potential interventions, DTA helps us deliver superior solutions. Our teams are actively evaluating different DTA packages and seeking opportunities to integrate them more comprehensively into our projects, enhancing our overall modeling capabilities and the effectiveness of corridor studies.
How will advances in AI and machine learning affect travel model work?
At RSG, we are exploring and looking for ways to integrate the vast potential of AI tools and technologies through our AI Steering Committee and company-wide strategic initiatives. In the context of our travel model development work, it is important to recognize the foundational role of machine learning, which we have extensively used for data processing and imputation. Recently, there has been interest in using machine learning models to replace traditional choice models for modeling travel behaviors. However, a significant challenge with AI models is their lack of transparency. Our clients often require a clear understanding of how inputs are transformed into results, a process that can be less transparent with AI.
Nevertheless, AI offers substantial benefits in enhancing our documentation capabilities and streamlining the model calibration and validation process by optimizing adjustments and reducing discrepancies in traffic counts, for example. Despite the advantages of AI, maintaining transparency in these processes is crucial to control and promote understanding of changes. At RSG, striking a balance between leveraging AI's capabilities and ensuring transparency remains a key focus of ours, guiding our decisions on how we develop our models to best serve our clients and communities.
·····························
Joel Freedman is a nationally recognized leader in the development and application of advanced travel demand forecasting models. He has developed travel demand models for some of the largest and most complex metropolitan regions in the United States and large-scale integrated models of land use and transportation for state departments of transportation. Joel works closely with RSG’s household travel survey teams to ensure an accurate and relevant data pipeline for modeling purposes.
Have questions about an upcoming travel model development project? Contact us to request a consultation.